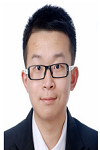
Yao Yao
Sun Yat-sen University
China
Title: Extracting urban impervious surface from GF-1 imagery based on one-class classifiers
Biography
Biography: Yao Yao
Abstract
Impervious surface area is a direct consequence of the urbanization, which also plays an important role in urban planning and environmental management. With rapid technique development of remote sensing, Monitoring urban impervious surface via high spatial resolution (HSR) images has attracted unprecedented attention recently. Traditional multi-classes models are inefficient for impervious surface extraction because it requires labeling all known and unknown classes that occur in the image exhaustively, so we need to find a reliable one-class model to classify one specific land cover type without labeling other classes. In this study, we investigate several one-class classifiers, such as Presence and Background Learning (PBL), Positive Unlabeled Learning (PUL), OCSVM, BSVM and MAXENT, to extract urban impervious surface area using high spatial resolution imagery of GF-1, China’s new generation of high spatial remote sensing satellite, and evaluate the classification accuracy based on artificial interpretation results. Compared to traditional multi-classes classifiers (ANN and SVM), the experimental results indicate that PBL and PUL provide highest classification accuracy, which is similar to the accuracy provided by ANN model. More surprisingly, it outperforms OCSVM, BSVM, MAXENT and SVM models. Hence, the one-class classifiers only need a small set of specific samples to train models without losing predictive accuracy.